Evaluation of the effectiveness of PCA and ICA in improving muscle movement recognition from raw EMG signals
DOI:
https://doi.org/10.37636/recit.v6n4e318Keywords:
EMG, Cross communication, Machine Learning, Data miningAbstract
In the last decade, the development of classification models through machine learning for the control of multifunctional prosthetic devices has been increasing. Electromyography (EMG) are recordings produced by muscle fibers naturally when performing movements; if modeled, they could play a more active role in this type of control. These signals are used to control devices/applications. The problem with these models is the stochastic nature of the signal, the variability between subjects and the inherent cross-communication that makes them inaccurate when faced with a high number of movements. The stochastic nature and variability of the signal are already widely studied, however, there are still no definitive results that describe generalizable movement classification models. Here, two databases available on the CapgMyo network and the Ninapro project are studied, their characteristics are evaluated, with the objective of investigating the variability of the muscle signal between subjects, the factors that modify it and how the use of analysis affects principal components (PCA) and independent component analysis (ICA) to EMG information in classification models. A comparison was made between the results in terms of recognition percentages of classic machine learning methods such as linear discriminant analysis (LDA) and quadratic analysis (QDA) using transformation techniques to new spaces introducing the possibility of performing a dimensionality reduction. with PCA and ICA, algorithms usually used to solve problems such as blind source separation (BSS), which is applicable to the phenomenon presented in muscle signals and their acquisition through surface electrodes. The results can be evaluated through the recognition percentage of the classification models created, these show that for raw EMG signals the PCA and ICA methods are useful to perform a reduction in the dimensionality of the data without providing a significant increase in the recognition percentages. It was shown that the recognition percentages in the classification of movements for the Capgmyo database were higher thanks to the characteristics that define it, a higher recognition percentage was obtained ranging from 72.5% to 87.9% with QDA, and 82.8% to 90% for QDA with PCA. The main contribution is the evaluation of the effectiveness of algorithms such as PCA and ICA in machine learning tasks with raw EMG data. Future work is to lay the foundations to reduce the effects of cross-communication in EMG recordings.
Downloads
References
F. Stival, S. Michieletto, M. Cognolato, E. Pagello, H. Müller, and M. Atzori, “A quantitative taxonomy of human hand grasps,” Journal of NeuroEngineering and Rehabilitation, vol. 16, no. 1, p. 28, Feb. 2019, doi: 10.1186/s12984-019-0488-x. DOI: https://doi.org/10.1186/s12984-019-0488-x
Md. J. Islam et al., “Optimizing electrode positions on forearm to increase SNR and myoelectric pattern recognition performance,” Engineering Applications of Artificial Intelligence, vol. 122, p. 106160, Jun. 2023, doi: 10.1016/j.engappai.2023.106160. DOI: https://doi.org/10.1016/j.engappai.2023.106160
M. Beretta-Piccoli, C. Cescon, M. Barbero, and G. D’Antona, “Identification of muscle innervation zones using linear electrode arrays: a fundamental step to measure fibers conduction velocity,” Arab Journal of Basic and Applied Sciences, vol. 28, no. 1, pp. 264–271, Jan. 2021, doi: 10.1080/25765299.2021.1894731. DOI: https://doi.org/10.1080/25765299.2021.1894731
N.V. Thakor, “Biopotentials and Electrophysiology Measurements” in Telehealth and Mobile Health. CRC Press, (2015), https://doi.org/10.1201/b19147-36 DOI: https://doi.org/10.1201/b19147-36
J. Amezquita-Garcia, M. Bravo-Zanoguera, F. F. Gonzalez-Navarro, R. Lopez-Avitia, and M. A. Reyna, “Applying Machine Learning to Finger Movements Using Electromyography and Visualization in Opensim,” Sensors, vol. 22, no. 10, 2022, doi: 10.3390/s22103737. DOI: https://doi.org/10.3390/s22103737
Y. Du, W. Jin, W. Wei, Y. Hu, and W. Geng, “Surface EMG-Based Inter-Session Gesture Recognition Enhanced by Deep Domain Adaptation,” Sensors, vol. 17, no. 3, 2017, doi: 10.3390/s17030458 DOI: https://doi.org/10.3390/s17030458
Marco Santello, Martha Flanders, and John F. Soechting, “Postural Hand Synergies for Tool Use,” J. Neurosci., vol. 18, no. 23, p. 10105, Dec. 1998, doi: 10.1523/JNEUROSCI.18-23-10105.1998. DOI: https://doi.org/10.1523/JNEUROSCI.18-23-10105.1998
D. Buongiorno et al., “Deep learning for processing electromyographic signals: A taxonomy-based survey,” Neurocomputing, vol. 452, pp. 549–565, Sep. 2021, doi: 10.1016/j.neucom.2020.06.139. DOI: https://doi.org/10.1016/j.neucom.2020.06.139
E. Ayodele, S. A. R. Zaidi, Z. Zhang, J. Scott, and D. McLernon, “Chapter 9 - A review of deep learning approaches in glove-based gesture classification,” in Machine Learning, Big Data, and IoT for Medical Informatics, P. Kumar, Y. Kumar, and M. A. Tawhid, Eds., Academic Press, 2021, pp. 143–164. doi: 10.1016/B978-0-12-821777-1.00012-4. DOI: https://doi.org/10.1016/B978-0-12-821777-1.00012-4
R. Donati, V. Kartsch, L. Benini, and S. Benatti, “BioWolf16: a 16-channel, 24-bit, 4kSPS Ultra-Low Power Platform for Wearable Clinical-grade Bio-potential Parallel Processing and Streaming,” in 2022 44th Annual International Conference of the IEEE Engineering in Medicine & Biology Society (EMBC), Jul. 2022, pp. 2518–2522. doi: 10.1109/EMBC48229.2022.9871898. DOI: https://doi.org/10.1109/EMBC48229.2022.9871898
P. Huang et al., “Identification of Upper-Limb Movements Based on Muscle Shape Change Signals for Human-Robot Interaction,” Computational and Mathematical Methods in Medicine, vol. 2020, p. 5694265, Apr. 2020, doi: 10.1155/2020/5694265. DOI: https://doi.org/10.1155/2020/5694265
Y. A. Jarrah et al., “High-density surface EMG signal quality enhancement via optimized filtering technique for amputees’ motion intent characterization towards intuitive prostheses control,” Biomedical Signal Processing and Control, vol. 74, p. 103497, Apr. 2022, doi: 10.1016/j.bspc.2022.103497. DOI: https://doi.org/10.1016/j.bspc.2022.103497
R. Soangra, R. Sivakumar, E. R. Anirudh, S. V. Reddy Y., and E. B. John, “Evaluation of surgical skill using machine learning with optimal wearable sensor locations,” PLOS ONE, vol. 17, no. 6, p. e0267936, Jun. 2022, doi: 10.1371/journal.pone.0267936. DOI: https://doi.org/10.1371/journal.pone.0267936
A. Maheen et al., Human Hand Gesture Recognition System Using Body Sensor Network. 2021, p. 5. doi: 10.1109/ICRAI54018.2021.9651389. DOI: https://doi.org/10.1109/ICRAI54018.2021.9651389
R. Esaa, H. jaber, and A. A. Jasim, “Features selection for estimating hand gestures based on electromyography signals,” Bulletin of Electrical Engineering and Informatics, vol. Vol. 12, pp. 2087–2094, Aug. 2023, doi: 10.11591/eei.v12i4.5048. DOI: https://doi.org/10.11591/beei.v12i4.5048
M. Aviles, L.-M. Sánchez-Reyes, R. Q. Fuentes-Aguilar, D. C. Toledo-Pérez, and J. Rodríguez-Reséndiz, “A Novel Methodology for Classifying EMG Movements Based on SVM and Genetic Algorithms,” Micromachines, vol. 13, no. 12, 2022, doi: 10.3390/mi13122108. DOI: https://doi.org/10.3390/mi13122108
B. Saeed et al., “Leveraging ANN and LDA Classifiers for Characterizing Different Hand Movements Using EMG Signals,” Arabian Journal for Science and Engineering, vol. 46, no. 2, pp. 1761–1769, Feb. 2021, doi: 10.1007/s13369-020-05044-x. DOI: https://doi.org/10.1007/s13369-020-05044-x
Rami N. Khushaba, “Electromyogram (EMG) Data, 8 Channels, 15 Classes.” [Online]. Available: https://www.rami-khushaba.com/biosignals-repository
P. Kaczmarek and J. Tomczyński, “putEMG: sEMG Gesture and Force Recognition Datasets.” 2019. [Online]. Available: https://biolab.put.poznan.pl/putemg-dataset/
C. Sapsanis, A. Tzes, and G. Georgoulas, “sEMG for Basic Hand movements.” UCI Dataset, Nov. 17, 2014. [Online]. Available: https://archive.ics.uci.edu/dataset/313/semg+for+basic+hand+movements
A. Balbinot and T. Oliveira Weber, “IEE EMG Database.” [Online]. Available: https://www.ufrgs.br/ieelab/resource_IEE_sEMG_db.php
K. Zhao et al., “Evaluation of Methods for the Extraction of Spatial Muscle Synergies,” Frontiers in Neuroscience, vol. 16, 2022, doi: 10.3389/fnins.2022.732156. DOI: https://doi.org/10.3389/fnins.2022.732156
N. J. Jarque-Bou, A. Scano, M. Atzori, and H. Müller, “Kinematic synergies of hand grasps: a comprehensive study on a large publicly available dataset,” Journal of NeuroEngineering and Rehabilitation, vol. 16, no. 1, p. 63, May 2019, doi: 10.1186/s12984-019-0536-6. DOI: https://doi.org/10.1186/s12984-019-0536-6
G. Weidong, “CapgMyo: A High Density Surface Electromyography Database for Gesture Recognition.” 2017. [Online]. Available: http://zju-capg.org/research_en_electro_capgmyo.html
M. Atzori, A. Gijsberts, and C. Castellini, et al, “DB2 - 40 Intact Subjects - Delsys Trigno electrodes.” [Online]. Available: http://ninaweb.hevs.ch/node/17
M. Atzori et al., “Characterization of a Benchmark Database for Myoelectric Movement Classification,” IEEE Transactions on Neural Systems and Rehabilitation Engineering, vol. 23, no. 1, pp. 73–83, Jan. 2015, doi: 10.1109/TNSRE.2014.2328495. DOI: https://doi.org/10.1109/TNSRE.2014.2328495
G. Masri, H. Harb, N. Diab, and R. Halabi, “Design and Control of a Myoelectric Prosthetic Hand using Multi-Channel Blind Source Separation Techniques,” in 2021 Sixth International Conference on Advances in Biomedical Engineering (ICABME), Oct. 2021, pp. 54–58. doi: 10.1109/ICABME53305.2021.9604876. DOI: https://doi.org/10.1109/ICABME53305.2021.9604876
A. Tharwat, “Independent component analysis: An introduction,” Applied Computing and Informatics, vol. 17, no. 2, pp. 222–249, Jan. 2021, doi: 10.1016/j.aci.2018.08.006. DOI: https://doi.org/10.1016/j.aci.2018.08.006
A. Sultana, F. Ahmed, and Md. S. Alam, “A systematic review on surface electromyography-based classification system for identifying hand and finger movements,” Healthcare Analytics, vol. 3, p. 100126, Nov. 2023, doi: 10.1016/j.health.2022.100126. DOI: https://doi.org/10.1016/j.health.2022.100126
L. Dela, D. Sutopo, S. Kurniawan, T. Tjahjowidodo, and W. Caesarendra, “EMG Based Classification of Hand Gesture Using PCA and SVM,” in Proceedings of the 2nd International Conference on Electronics, Biomedical Engineering, and Health Informatics, T. Triwiyanto, A. Rizal, and W. Caesarendra, Eds., Singapore: Springer Nature Singapore, 2022, pp. 459–477. DOI: https://doi.org/10.1007/978-981-19-1804-9_35
R. Howard, R. Conway, and A. Harrison, The use of Independent Component Analysis on EMG Data to Explore Cross-Talk. 2015.
Yonghong Huang, K. B. Englehart, B. Hudgins, and A. D. C. Chan, “A Gaussian mixture model based classification scheme for myoelectric control of powered upper limb prostheses,” IEEE Transactions on Biomedical Engineering, vol. 52, no. 11, pp. 1801–1811, Nov. 2005, doi: 10.1109/TBME.2005.856295. DOI: https://doi.org/10.1109/TBME.2005.856295
Y. Chen et al., “A hierarchical dynamic Bayesian learning network for EMG-based early prediction of voluntary movement intention,” Scientific Reports, vol. 13, no. 1, p. 4730, Mar. 2023, doi: 10.1038/s41598-023-30716-7. DOI: https://doi.org/10.1038/s41598-023-30716-7
D. Berrar, “Cross-Validation,” in Encyclopedia of Bioinformatics and Computational Biology, S. Ranganathan, M. Gribskov, K. Nakai, and C. Schönbach, Eds., Oxford: Academic Press, 2019, pp. 542–545. doi: 10.1016/B978-0-12-809633-8.20349-X. DOI: https://doi.org/10.1016/B978-0-12-809633-8.20349-X
W. Geng, Y. Du, W. Jin, W. Wei, Y. Hu, and J. Li, “Gesture recognition by instantaneous surface EMG images,” Scientific Reports, vol. 6, no. 1, p. 36571, Nov. 2016, doi: 10.1038/srep36571. DOI: https://doi.org/10.1038/srep36571
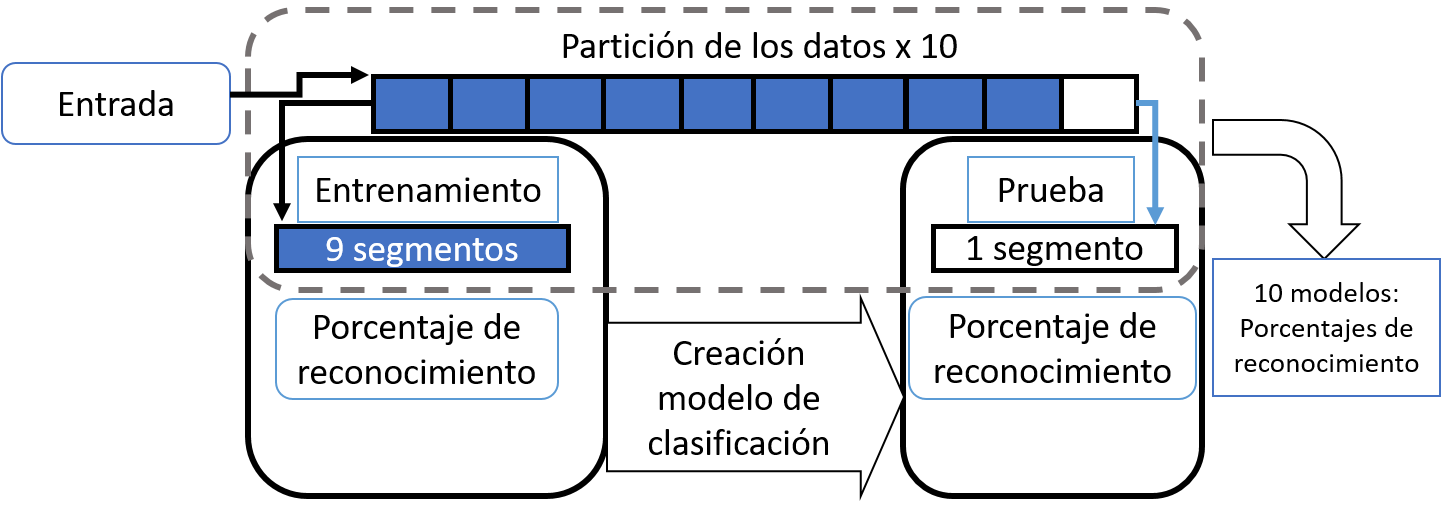
Published
How to Cite
Issue
Section
Categories
License
Copyright (c) 2023 José Alejandro Amézquita García, Miguel Enrique Bravo Zanoguera, Fabián Natanael Murrieta-Rico

This work is licensed under a Creative Commons Attribution 4.0 International License.
The authors who publish in this journal accept the following conditions:
The authors retain the copyright and assign to the journal the right of the first publication, with the work registered with the Creative Commons Attribution license 4.0, which allows third parties to use what is published as long as they mention the authorship of the work and the first publication in this magazine.
Authors may make other independent and additional contractual agreements for the non-exclusive distribution of the version of the article published in this journal (eg, include it in an institutional repository or publish it in a book) as long as they clearly indicate that the work it was first published in this magazine.
Authors are allowed and encouraged to share their work online (for example: in institutional repositories or personal web pages) before and during the manuscript submission process, as it can lead to productive exchanges, greater and more quick citation of published work (see The Effect of Open Access).